Personal projects
These are examples of the kinds of projects ive done in my free time. for fun, practice or just because it something i really want to do.
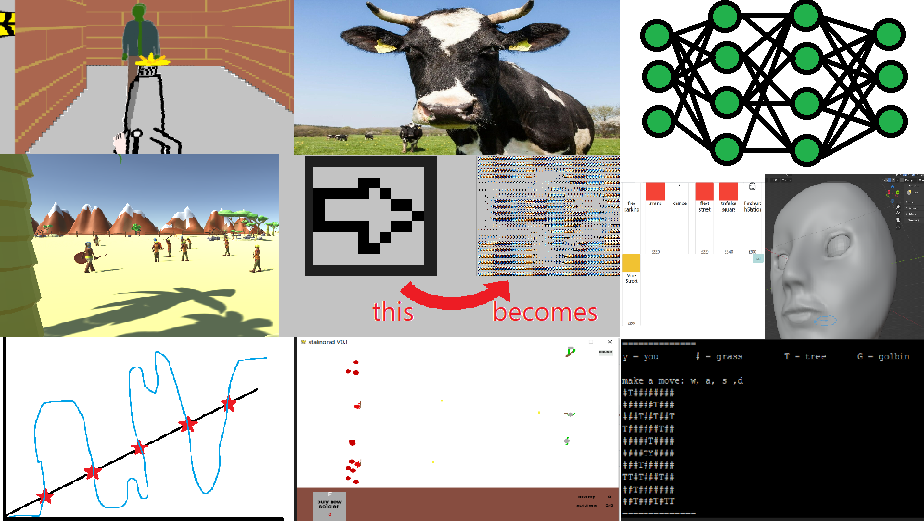
Early days of coding
From a young age I used animation software starting with frame by frame style flip book stuff, working my way up to flash by the time I was 12/13. and i had always wanted to make my own games I started learning to code between my first and second year at university. I began just learning python using pygame to make little 2d games by following whatever tutorials i could find. i did this for fun and practice. below are a few examples of games made in my early days.
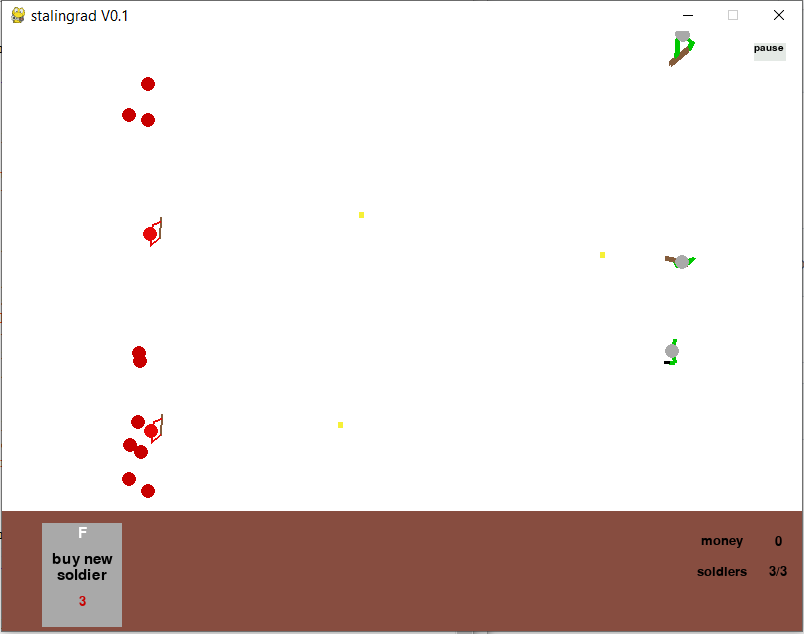
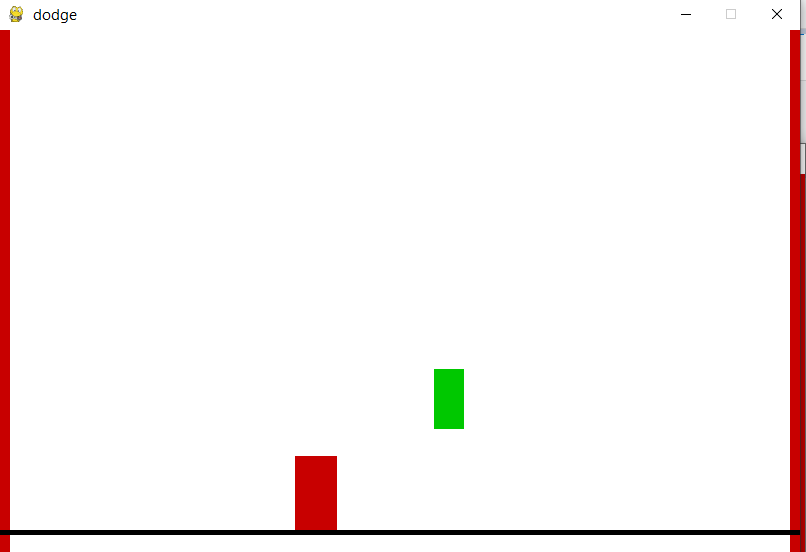
A first person shooter in JavaScript from scratch
One example of a project I’ve worked on in my own time is this first person shooter (click anywhere in this section to play it). I began learning 3d programming methods a while ago, I started with view frustums from scratch which taught me many applications of matrices that my education hadn’t covered, and building view frustums made me want to see if I could build a first person shooter from scratch, with no help from powerful tools like unity of 3d graphics libraries. after trying for a while with a view frustum (not realizing at the time that tessellation was a necessary step to use a view frustum in this way, rather than just a nice extra to cut down on unnecessary rendering) i switched to recasting methods for the graphics. This game is like a cod zombies style survival shooter.
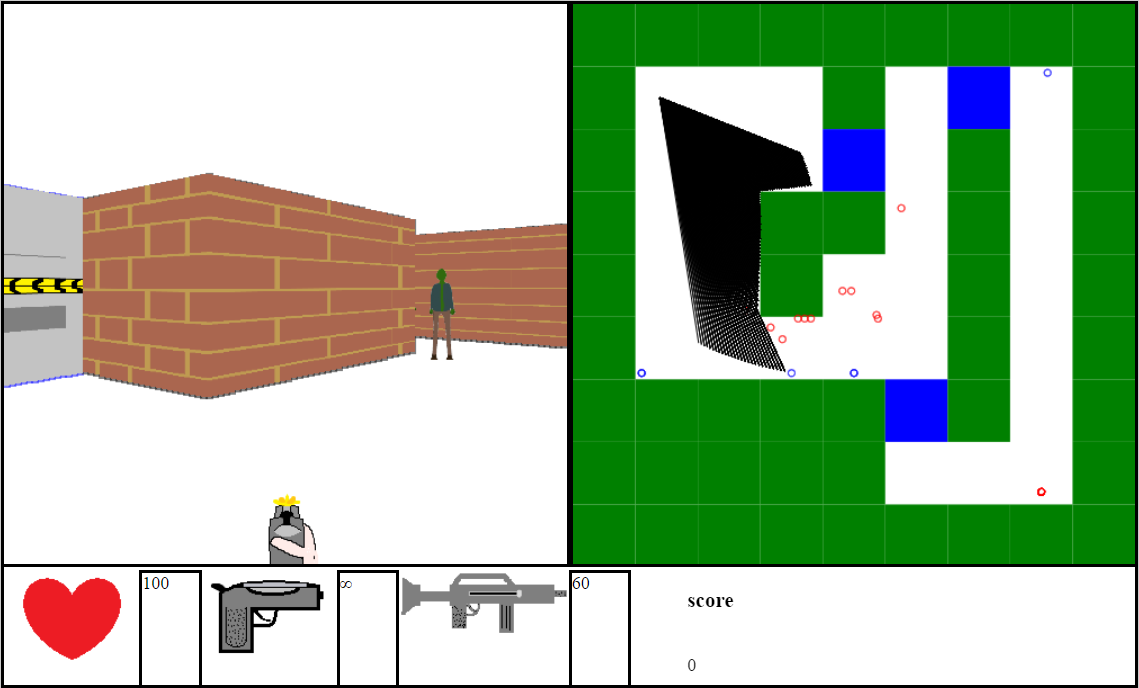
Websites
Django
I've built many systems with Django including basic front end systems to tie together projects both professionally and personally and my personal Websites, one of which youre currently viewing
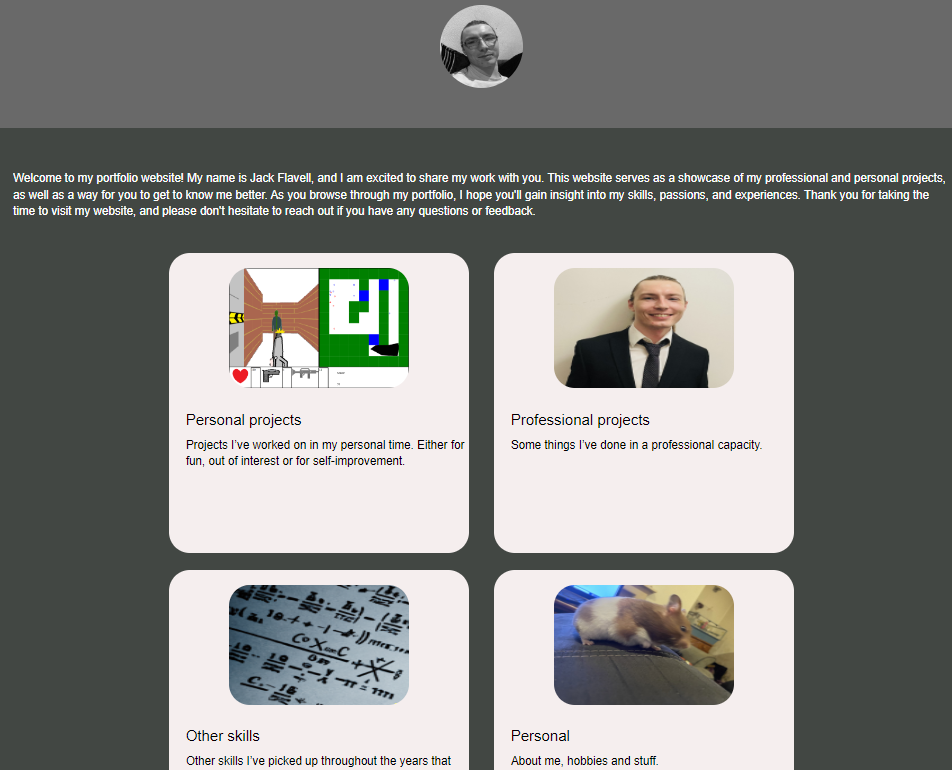
Other
I've been working on a game of monopoly in react. I have components for the tiles, dice rolls, cards and other parts all fiished. It just requires a lot of prettying up and a decent AI to play against and it will be finished. Click here to see my project.
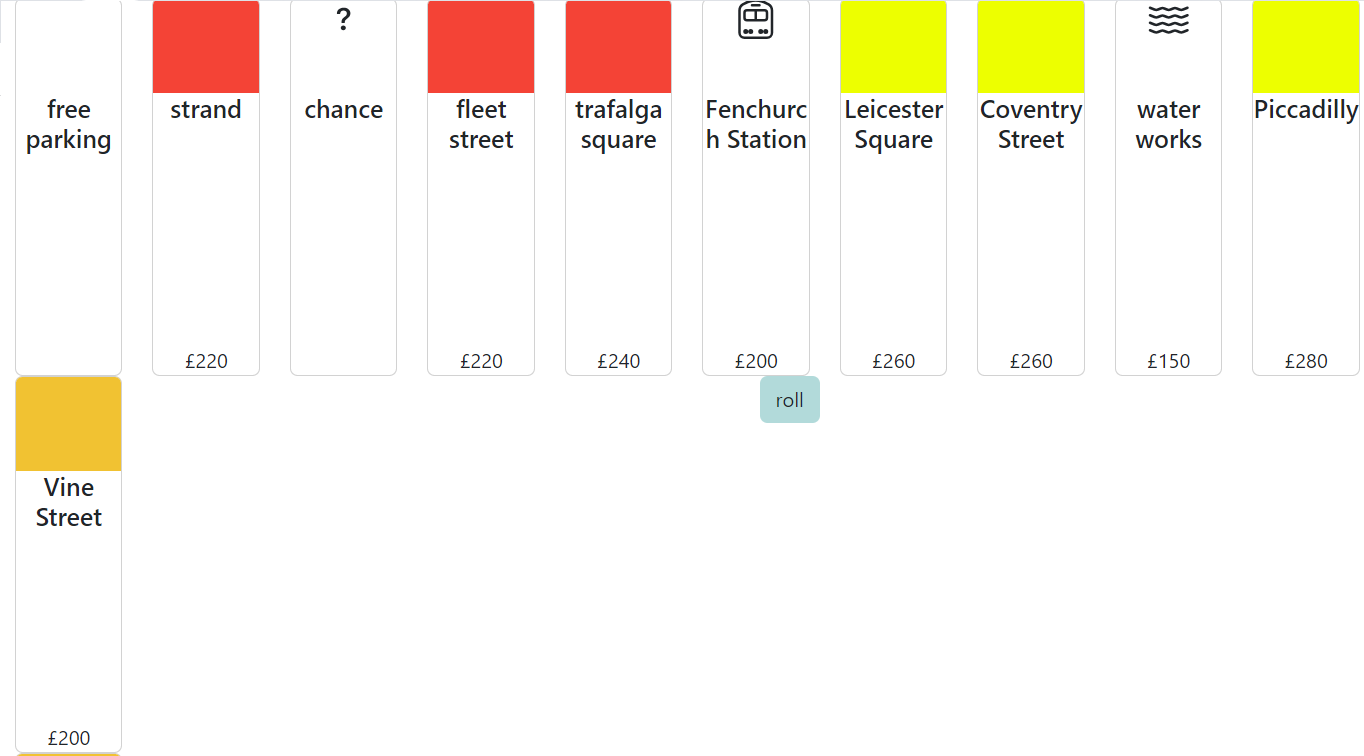
Finally, when I first began learning web technologies several years ago, I was mostly using MVC systems like .NET and am generally acustomed with MVC's.
Other
Equation reverse engineerer
It does what it says on the tin. I built a genetic algorithm which essential evolves an equation until it describes the data the user fed into it. on one level technically it worked in the end, on another level it technically didn't. it does produce an equation which describes the data you feed into it. however, that doesn’t mean it can predict unseen data There are an infinite number of arbitrary solutions to every problem. i.e. A = A & A = A + 1 - 1 & A = A + 2 - 2 & .... etc.... so, if you use suvat data I intended for it to be capable of returning v = u+at for example. but the odds of that are basically zero, it will simply come up with any equation that describes the data its being shown.
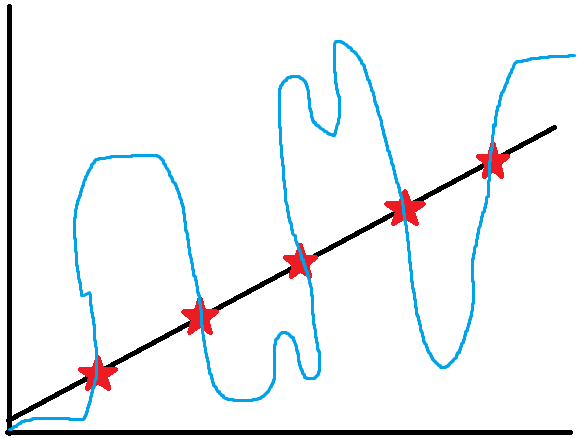
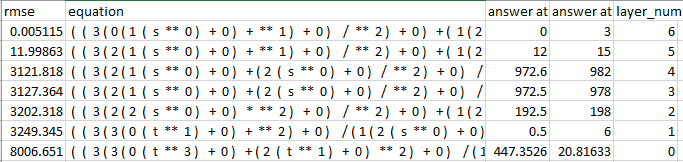
Unity and blender
working with unity and blender (and sometimes over software like vroid) I have made games and all of the assets within them. Aside from practice with C#, and a deeper knowledge of animation/ game making / rigging and modelling working with unity also caused me to learn topics like quaternions, throughout my official education I only really knew about the first dimension of imaginary units (i). So using unity caused me to gain a greater understanding of complex numbers.
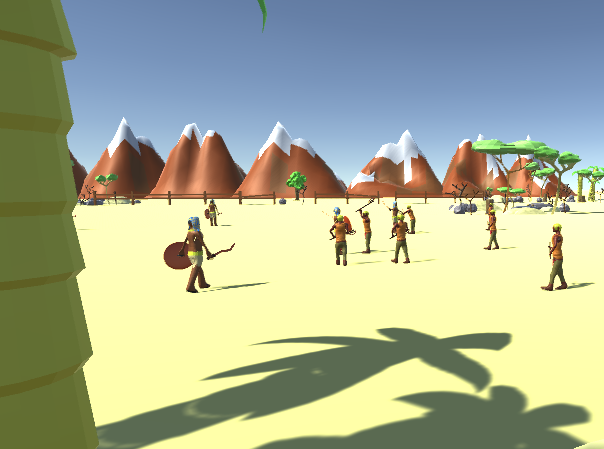
Misc
There’s lots of other little projects I could point to too, like these 3d graphics systems made from scratch or this little turn based rpg made with shell scripting, to be played in a command line. Check my github for more.
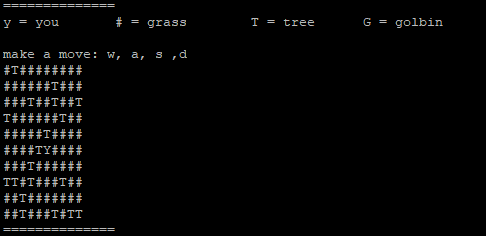
Machine learning
I started practicing machine learning early on. For an early project i started training agents to play simple games. for example, I made a very simple platformer (see jumper at top of page). using LSTM to train the agent to avoid incoming obstacles
In another project I built an automated categorizer, the user provides a list of 2 words (ideally concrete nouns) and then web scrappers automatically start collecting related images from google. Once the data is collected, its separated into training and testing data, and then a CNN is trained to differentiate between those 2 groups of pictures. finally, the system is tested against the testing data to calculate accuracy. this system can easily get well over 90% accuracy, from a couple hundred pictures (5 minutes of scraping), and 2 minutes of training. Link to automated categorizer, try it yourself.
As well as just categorizers i have built bounding box systems which locate a specific object in an image. Collecting my own data to train these systems was far too time consuming however as it couldnt be done in an automated fashion.
I have attempted several Kaggle competitions, for example one which involved the detection of gravity waves. While I learned much both in machine learning, and more widely applicable subjects through doing this, I found I had the same problem. that I had with unity, that after a while of working it became apparent that months of work would be required for me to benefit and I really had to work on other things to try to make more money. I also intend to return to Kaggle soon.
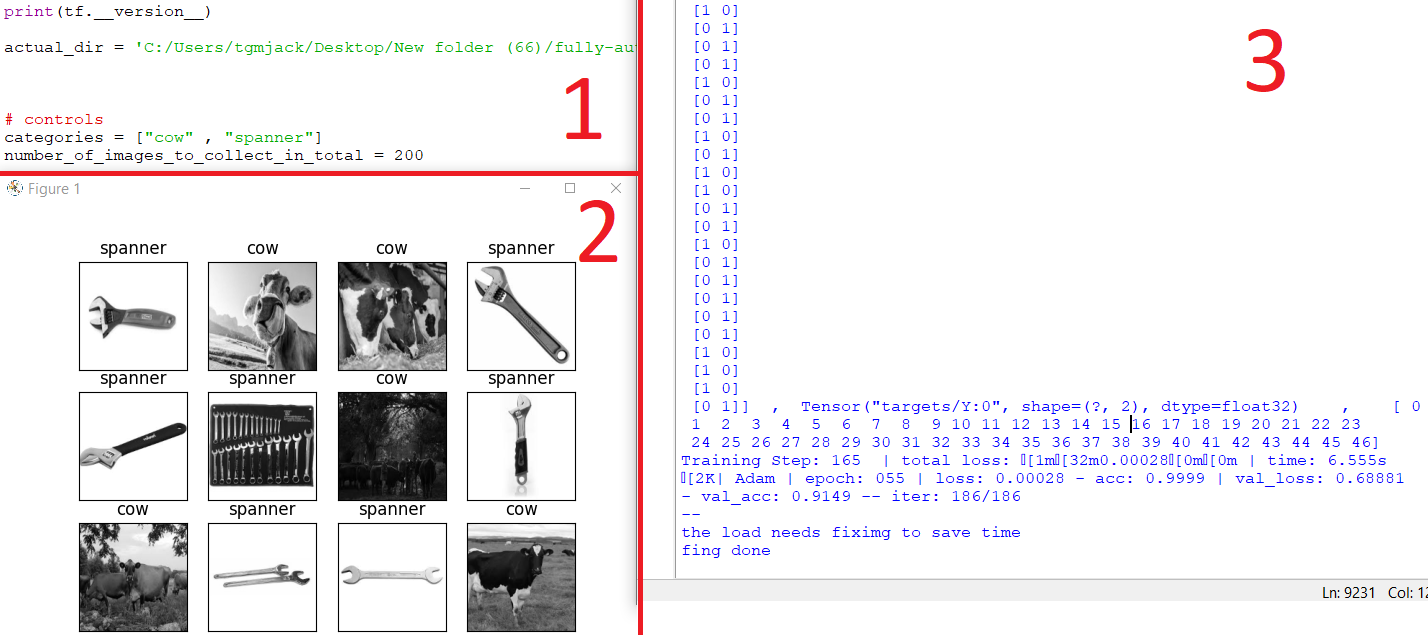
The image above is a demonstration of the automated categorizer. the image is in 3 pieces.
1) shows a small section of the top of the code. the user simply enters 2 words (ideally concrete nouns) into the list named categories. these are the 2 types of images that will be collected by the webscrappers and the model will be trained to differentiate between.
2) shows a matplotlib window which is used to demonstrate the final ability of the categorizer. the word above each image is the category the model believes the image is.
3) is a section of the end output from the script. An import part of this output is "val_acc: 0.9149" showing that the model is more than 91% accurate. which considering a rather minimal amount of training time and data used, isn't bad.